Authors: Muhammad Rafi Bakri and Rio Tirta, SAI of Indonesia
Introduction
Big Data Analytics (BDA) has increased significantly in recent years. BDA is frequently used to represent immense amounts of data, so users can analyze it more efficiently and make judgements in audit process (Saggi and Jain 2018). Consequently, the worldwide auditing community has formed to mobilize the use of BDA in auditing (Appelbaum, Kogan, and Vasarhelyi 2017).
INTOSAI, as the worldwide supreme audit institution (SAI) organization, started the BDA movement by forming the INTOSAI Working Group on Big Data (WGBD) in December 2016. The establishment of INTOSAI WGBD aligns with Strategic Goal III, which aims to increase SAI capabilities worldwide in the big data era.
Based on the INTOSAI WGBD Development Overview (INTOSAI 2022a), at least 9 SAIs have introduced big data audit infrastructure such as China, Russia, Brazil, Norway, Estonia, Turkey, and the Philippines. Moreover, the European Court of Auditors has also erected a big data audit platform that EU countries can utilize in 2021. SAI of Indonesia, known as the “Badan Pemeriksa Keuangan (BPK)”, was an early adopter of BDA by introducing a big data audit platform in 2017. BPK’s platform, BPK Big Data Analytics, or BIDICS, can be used as a forum for collecting, processing, and conducting various data analysis according to audit needs (INTOSAI 2022c).
E-Audit: The Origin of BIDICS
BIDICS is a platform that assists the BPK audit process by utilizing BDA. Before becoming a platform, BIDICS had a more old-school form called e-Audit. E-Audit,developed from 2010-2014, ussed the Collect-First concept, where BPK needed to collect the necessary data first and then validate it. Once the data is valid, the data can be used for audit analysis. The E-Audit format is the foundation to the formation of a data-driven organization.
E-Audit requires synergy between BPK and auditees in terms of data collection. BPK enters into an agreement with the auditee to request data related to financial reports so that it can be entered into the database. In 2014, BPK entered into 767 memorandums of understanding (MoU) with state institutions (auditees) to collect large amounts of data.
After the data is collected, BPK will re-validate the data. When the data is validated, BPK’s auditors can scrutinise all financial data from state institutions more quickly, easily and effectively. The use of e-Audit also makes audit activities more adequate and efficient. The procedure for collecting and using big data in the e-Audit application follows the technical pedagogy issued by INTOSAI (2022b) in 2022.
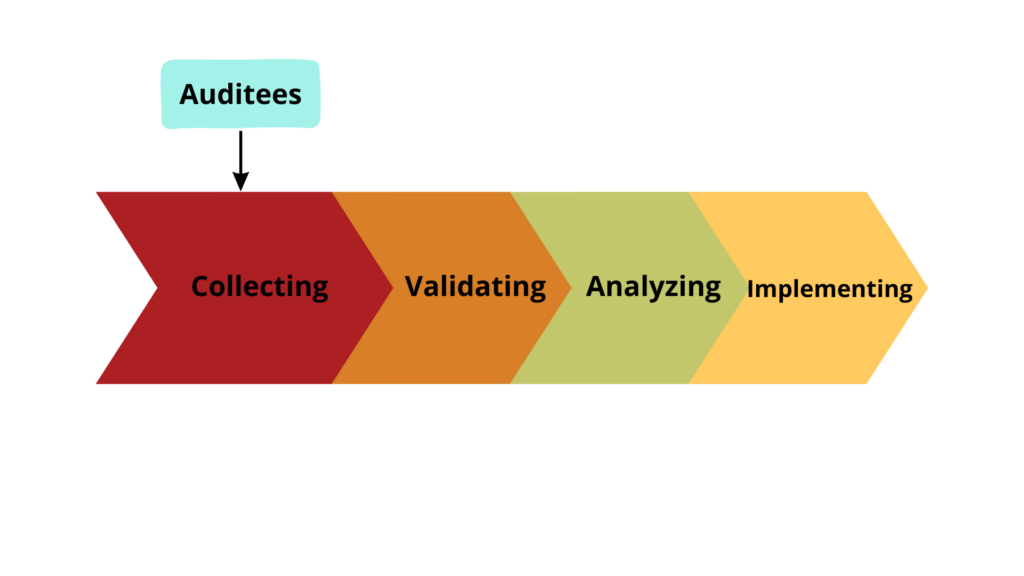
The implementation of e-Audit has a positive result on state finances. As of 2014, BPK could review 46,586 accounts, of which 19.95% were successfully sealed. Closing these accounts saw a saving of USD 11.8 million or IDR 7 trillion in state funds. These results made all of BPK’s internal and external parties more aggressive in evolving this platform and encouraging the broader use of BDA in audits.
BIDICS Enactment Framework
BPK Secretary General Decree Number 206/K/X-XIII.2/8/2021 underlies the grand design in implementing and developing BIDICS. The following figure shows the appointment and plot of enforcing BIDICS within the BPK circumstances (2021a).
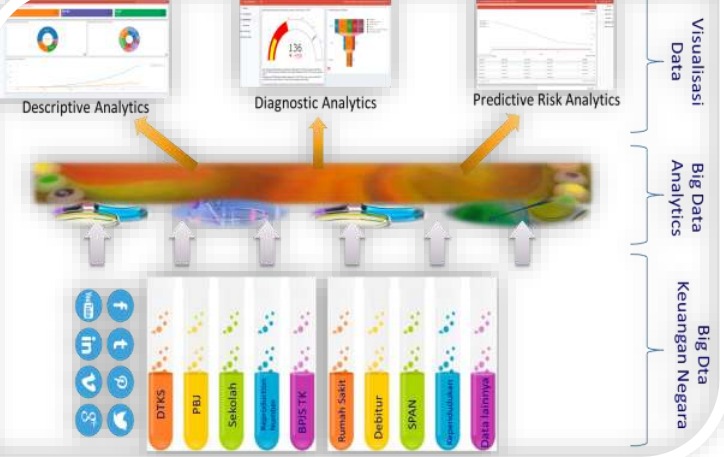
This scheme shows that BIDICS is a device for performing three types of analysis: descriptive, diagnostic, and risk prediction. These three analyses can demonstrate a pattern from the existing data set. Auditors can use these results to assist in inspections.
Two activities are interconnected in applying BIDICS, namely exploratory and confirmatory. Exploratory activities aim to find hints or signals of an anomaly, irregularity, or pattern of events. The information obtained through exploratory activities is then followed up to a confirmatory procedure to ensure that the results of the exploratory activities are sufficient enough to become audit evidence that is accurate, relevant, and competent. These two activities are the perfect combination for the auditor to conduct the audit.
The application of BIDICS uses a goal-based approach. In practice, auditors use BIDISC when a question arises. These questions are submitted to the BIDICS laboratory team to discuss the need for auditors and the availability of data to answer these questions.
The level of success in answering the auditor’s questions depends on data availability in the BIDICS database. This is the main background in applying the Collectability Over Validity principle by BIDICS. This principle emphasizes collecting as much data as possible before validating it by the laboratory team when the data will be used. This principle gives BIDICS an extensive and diverse range of data that can meet the needs of auditors.
Different conditions ensue when the data required by the auditor is not available. The BIDICS labor team will execute a search for related data so that this deficiency makes BIDICS develop even more extensively. It can be concluded that BIDICS was created from a question that has no answer.
Based on the BIDICS Development Report (2021), BPK uses a performance measurement method called the North Star Metric. This method allows BIDICS to grow rapidly and without limits. The measurement of BIDICS performance is highly dependent on how many questions the auditors answer by the analytical model of the platform. Moreover, the North Star Metric will encourage other metrics to develop, namely (1) growth in data collected, (2) growth in connected data, (3) growth in the number of analytical models presented in the dashboard, and (4) growth in the number of analytical models used in line-of-business applications.
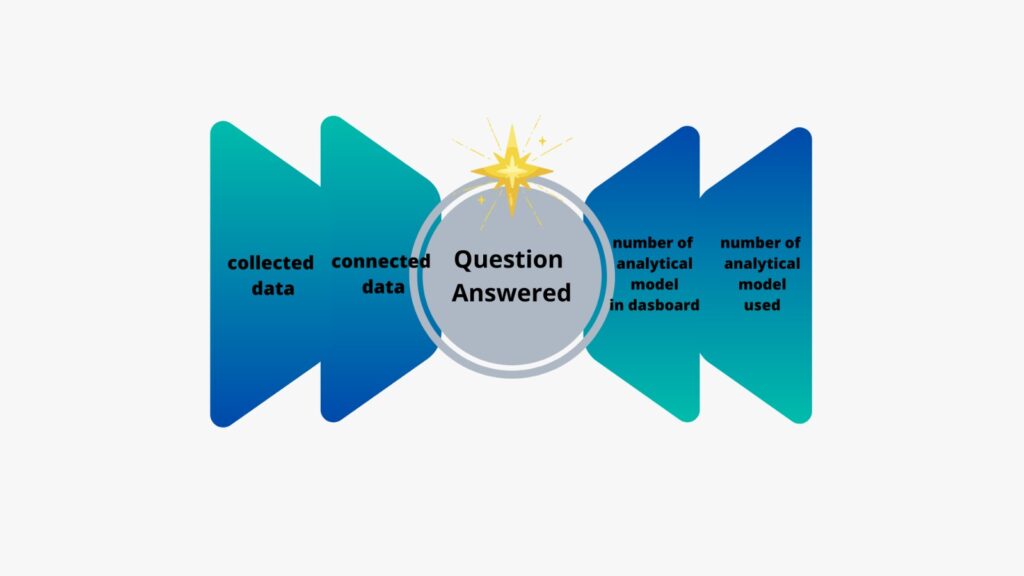
BIDICS Architecture
BIDICS performs an analysis of big data, which has very diverse variations. BIDICS can analyze structured, semi-structured and even unstructured data. Each of these types of data is commonly received by auditors when conducting audits. However, not all data received can be examined directly. For that, the role of BIDICS is beneficial here.
Structured data is the easiest to process because it is in the form of a table with rows and columns. Unstructured data is text, images, sound or video, so it does not have a clear pattern. The combination of these data is called semi-structured data. Examples of semi-structured data are data in the form of JavaScript Object Notation (JSON) files, Extensible Markup Language (XML) files, or HyperText Markup Language (HTML) files obtained from government websites or other organizations.
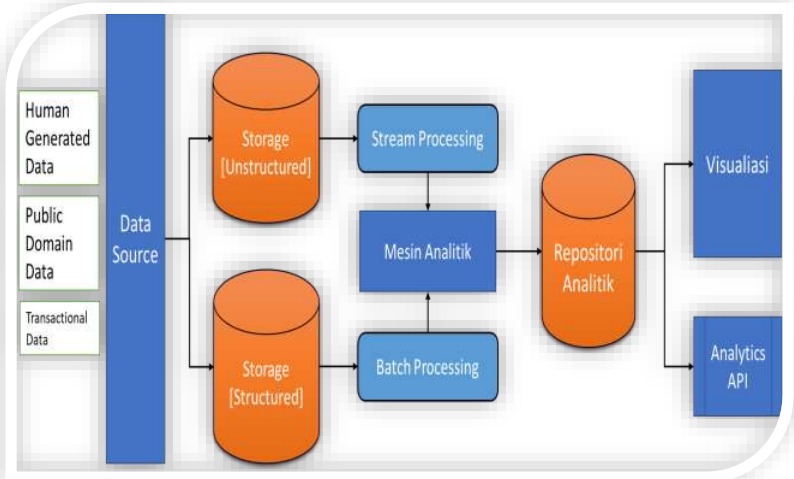
Structured data is processed through batch processing by an analytical engine, while unstructured data is processed through stream processing. This analytical engine then analyses based on statistical and mathematical algorithms. The analysis results are then stored in the analytical results repository to be displayed visually through a dashboard or consumed by applications commonly used by auditors.
The Application of BIDICS
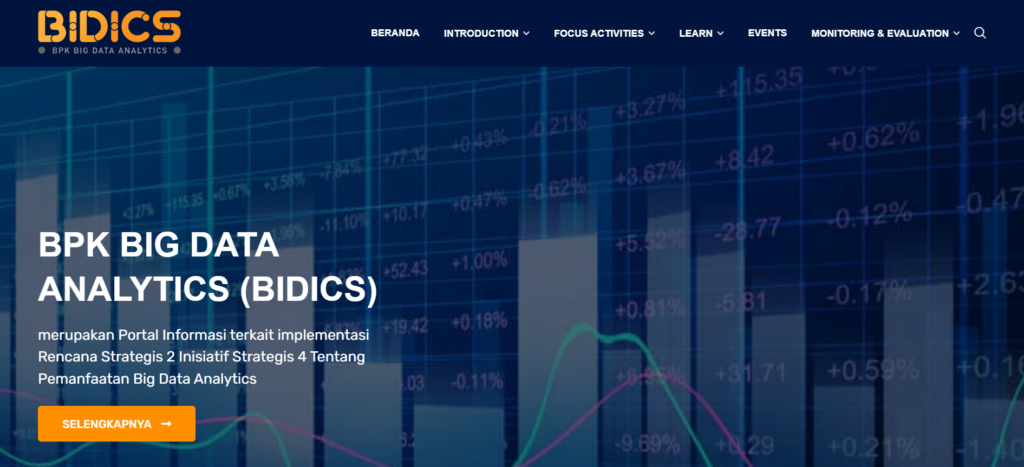
BIDICS is currently experiencing very significant evolution. BIDICS already has an intelligence dashboard that can be a medium for auditors to get to know the situation of the auditee, both financial and non-financial. The auditor can independently analyze the data from the available dashboard and answer the questions.
BIDICS can be accessed via https://bidics.bpk.go.id/ with a display that has several main menus, namely homepage, introduction, focus activities, learn, events, and monitoring & evaluation. Each existing menu has several sub-menus related to the introduction of BIDICS to the BIDICS report.
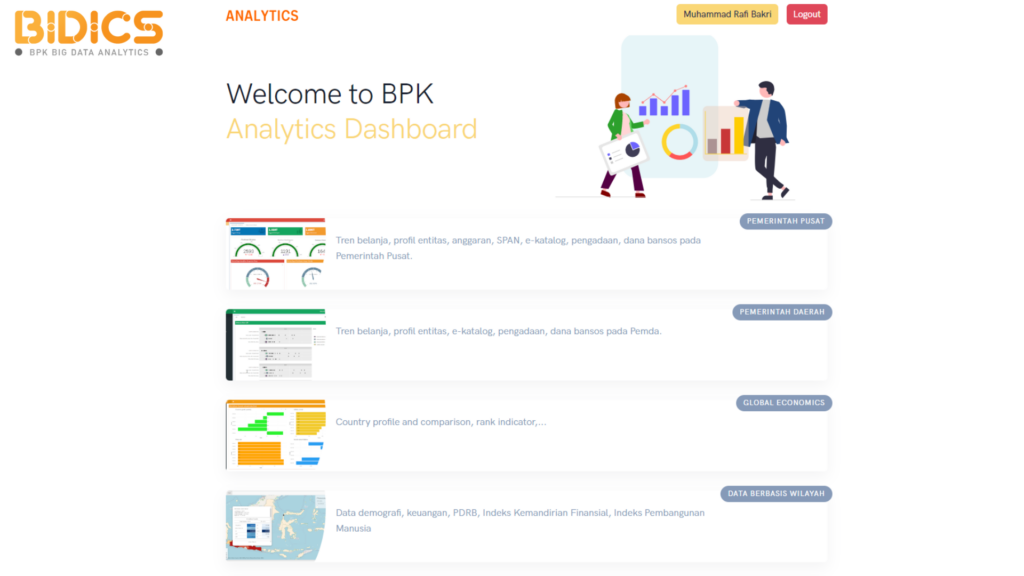
This condition transpires because the state financial big data in BIDICS is pervasive. As one of the prerequisites for facilitating the analytical process, Big Data on State Finance is equipped with a Data Catalog and implements Data Governance practices to increase reliability and confidence in optimal data utilization.
In addition, there is also a State Financial Analysis Laboratory whose function is to develop analytical models from BIDICS. This laboratory has an end-user computing function, a system for developing automation tools and making self-reporting. This laboratory is equipped with several workstations/computers with access to an analytical engine with sufficient computational power.
As of 2023, BIDICS has a total of 10 analytical data clusters that can be used in audits, namely central government, local government, global economy, area-based data, covid-19 data, social assistance and subsidies, national development maps, LPSE, search financial data, and institutional evaluation monitoring. These clusters have their sub-clusters, making it easier for the auditor to select the data to be used.
As part of BPK, BPK Jambi Province Representative has utilized BIDICS to reinforce audit activities executed on local governments. Auditors can see trends in
local government spending and revenues as part of audit planning. In addition, the auditor also can see the process of procuring goods and services and the e-catalog from the auditee within a particular period. Therefore, the auditor can measure the risk level of the auditee with low cost and fast time.
With the complexity of audit issues, BIDICS will continue develop. For this reason, BPK is collaborating with the University of Indonesia. For BPK, this collaboration adds insight into the story and utilization of BIDICS. In addition, BPK internally also formed special working groups from each work unit to develop BIDICS.
Conclusion
BPK has answered very well to challenges related to the flow of big data use in audits. Taking a long time, BPK succeeded in developing an analytical data-based audit platform called BIDICS. Today, BIDICS already has 10 data clusters that auditors can utilize in examinations. In the future, BIDICS will experience many challenges, given the increasingly complex audits conducted by BPK. For this reason, it is necessary to carry out unlimited development of BIDICS so that it continues to be helpful in audits.