Improve Budget Analysis With Machine Learning, Data Analytics
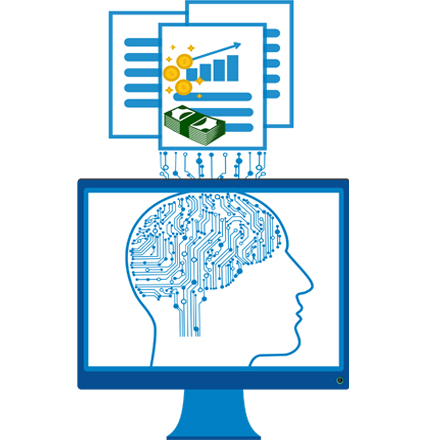
by Dr. Nunzio Mario Tritto, PhD., Magistrate at the Corte dei conti
Regional audit sections of Italy’s Corte dei conti, the nation’s Supreme Audit Institution (SAI), carry out various tasks, including analyzing local authority accounts to verify data accuracy and accounting system sustainability. The Apulia Region—consisting of 257 municipalities and other entities (such as local health authorities and universities) that must be audited annually—involves numerous accounts and annexes, which creates a very complicated analysis process, one traditionally carried out by the individual magistrate and his staff.
However, in recent years, specific legislative measures have been adopted requiring authorities to digitally enter relevant information into system that facilitates data collection, interpretation and examination. While the new system allows for enhanced data gathering, the national context (7,904 municipalities and hundreds of regional, provincial and metropolitan entities) makes analyzing all documents and data with due depth challenging.
To improve audit work, the Apulia region recently implemented particular mechanisms—advanced data analytic techniques and machine learning—that enable processing large volumes of information and analyzing massive quantities of data.
Without human intervention or assistance, machine learning, an artificial intelligence application, supplies system capabilities to automatically learn and improve based on experience. The learning process begins with observations (data)—seeking patterns and developing better decisions based on provided examples. The region’s system is designed to report deviations from benchmark parameters (established constraints indicating normalcy). As data is entered into the system, the software alerts staff to any anomalies found.
For example, Italian legislation dictates each municipality must maintain enough funds to meet any future expenses arising from ongoing litigation. As municipalities do not always make such provisions, they may lack necessary liquidity. By assessing these cases according to various risk-based criteria and giving each case appropriate, corresponding provisions, institutional volatility can be stabilized.
A similar analysis method has been applied to cash flow trends. As it is not unusual to see income and expenditure disparities, the data-driven approach is designed to alert auditors to situations potentially resulting in institutional liquidity shortfalls.
While data and human intelligence provide the foundation to audit work, the Corte dei conti has found advanced data analytics coupled with machine learning make it possible to improve audit work within the region, particularly in preventing crisis situations. The Apulia region’s system and techniques provide a platform for similar implementation efforts in regions across the nation.