Experiences on Auditing Algorithms and Artificial Intelligence in the Dutch Government
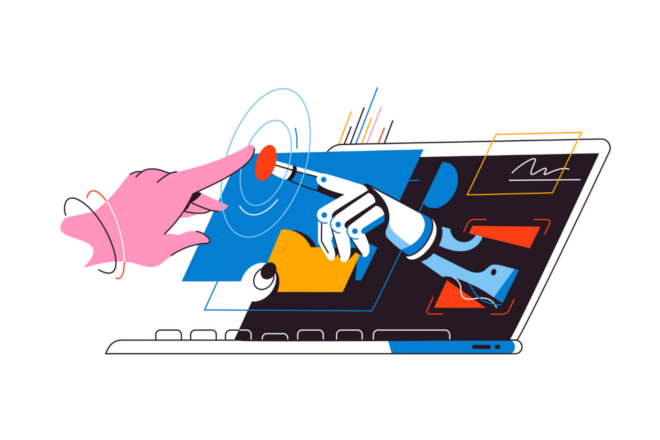
Authors: Colin van Noordt PhD, and Esther Meijer-van Leijsen PhD, Netherlands Court of Audit
Rationale of auditing algorithms and AI
The application of algorithms and artificial intelligence (AI) technology in government provides many opportunities for improving governmental processes, public service delivery, citizen engagement, and helping solve social challenges. As a result, this kind of technology is increasingly becoming a more important part of how governments operate. However, the introduction of AI also introduces risks if not deployed responsibly. For instance, AI might contain biases that lead to discriminatory outcomes or personal data may not be adequately protected. A lack of transparency when using the technology might lead to governance challenges.
Process of auditing algorithms
In 2021, the Netherlands Court of Audit (NCA) has developed an audit framework for algorithms. The framework covers both simple rule-based systems and more complex systems based on machine learning. It is a multidisciplinary framework, including norms on governance, privacy, model and data as well as general IT controls. In a previous article in this journal, we described the rationale and background behind the creation of the framework.
In 2022, we used this audit framework to audit nine algorithms used by the Dutch government. We found that 3 out of 9 audited algorithms met all the basic requirements. The other six did not and exposed the government to various risks: from inadequate control over the algorithm’s performance and impact to bias, data leaks and unauthorised access.
Since 2022 onwards, we audit AI as part of our annual audits. This allows us to better understand what these algorithms truly do, how the government ensures governance on their deployment and how negative consequences are avoided. This Spotlight contribution includes the practical experiences in doing so and provides several lessons learned.
Stepwise assessment of AI
We form our opinion of the use of algorithms and AI technologies as follows:
- Effectiveness of controls: We audit the effectiveness of all the controls included in our audit framework, based on the documentation submitted and conducted interviews. A control is assessed as ‘effective’, ‘partly effective’ or ‘ineffective’.
- Residual risk: We classify the residual risk as low, medium, or high. The residual risk is always high if the controls are ineffective. The risk classification may be lowered to either medium or low based on context and/or other supplementary measures.
- Conclusion: We then form our conclusion and determine if the use of the algorithm does or does not comply with the requirements set out in our audit framework.
- Final opinion: In case the algorithm does not comply with the audit framework, we decide whether to allocate a consideration or shortcoming to the minister. This is an overarching opinion.
Practical experiences with auditing algorithms
While governance norms are often rather general, these aspects are crosscutting across all the domains and are a central foundation in the audits. As noticed by one of our auditors:
“Often, when we identified issues in governance aspects during our audits, they also emerged in the other domains”.
For instance, if the performance of an algorithm is not monitored adequately, auditees often cannot provide evidence about risk mitigation at the model level of an algorithm as well. These risks might be amplified if the development and management of an algorithm is outsourced to a third party. However, in our view, working together with an external partner does not remove the responsibilities for controlling their algorithms from public administrations.
Our audits revealed a wide range of different privacy practices among government organisations. These range from organisations that completed extensive Data Protection Impact Assessments (DPIAs) and clearly defined data responsibilities to organisations that struggled to comply with legal requirements. The latter category often had a backlog of under-documented algorithms and limited resources to address this backlog. We found one organisation working to finalize over fifty different DPIAs with only a small team. There is also a variety in the depth of explanations to citizens with regard to the use of personal data. In some cases, we found only general information on websites, while in other cases a dedicated tool was available for citizens to gain this insight. On the bright side, we noticed a clear impact of our audits on these diverging practices:
“As a result of our audits, privacy has become a higher priority and organisations have made significant leaps in their data processing documentation after these audits”.
While auditing data and modelling aspects, we encountered two main issues. Firstly, there are currently no standardized ways of risk mitigation available, such as how to address biases or the choice of the models. Secondly, the development of the algorithms is regularly done in a silo environment. As a result, it may be challenging for business wishes to be conveyed to the development team. The opposite also occurs, for example when modelling decisions are not communicated in a manner that is actionable for that other specialists, such as those with a legal background or in management.
Our experiences auditing General IT Controls (GITC) show the importance of taking adequate time to explain the audit framework to IT administrators involved. Equally important is determining the scope of the audit object, such as the different IT components of the IT systems and the overall chain of service delivery. Determining this scope can help to identify the parties involved, and to analyse who is responsible for a specific component. However, most critically, the audit framework is only a tool and not a goal in itself. One of our team members stressed:
“No algorithm or AI system is the same, and adjustments to specificities, unique risks and needs might be required so that it can be applied purposefully and effectively.”
Despite the specialised domains during the auditing of algorithms, the complementarity of them together is key. As the project lead summarizes:
“Auditing AI requires a great deal of teamwork and the sharing of insights among each other. It is like different pieces of a puzzle coming together. Not one of the domains has the whole picture.”
Throughout the auditing process and the timeline, the importance of this complementarity should never be underestimated. A comprehensive audit of an algorithm requires all the perspectives to come together. Working together in a multidisciplinary team is an important prerequisite for a successful audit.
Impact and future perspectives
As a result of our audits, we have noticed a clear shift in the responsible use of AI systems in the Dutch government. Our audits have had a direct impact on the auditees, especially when shortcomings were detected. These organizations can have a wake-up call to mitigate the risks of their deployed AI systems more effectively. At the same time, we noticed a wider impact on the Dutch society. Our audit framework has been at the basis for additional guidelines for the responsible use of AI in the Netherlands, in both the public and the private sector. The special, independent and trustworthy role of a Supreme Audit Institution in this emerging topic thus has a noteworthy contribution to the governance landscape of AI.
As the field is still evolving, so are we. We are closely monitoring the developments in generative AI, as they will certainly influence governmental operations. Similarly, the AI Act of the European Commission will be applicable soon, introducing new rules on AI. Especially high-risk AI systems will be subject to several new legal requirements. Our audit framework will have to consider these aspects as well. However, even with these developments, it is important not to wait for this. AI systems are already in use now and our biggest advice is to just start auditing AI!