Understand How Disabled People Navigate Through the Residency Path with Optimal Matching
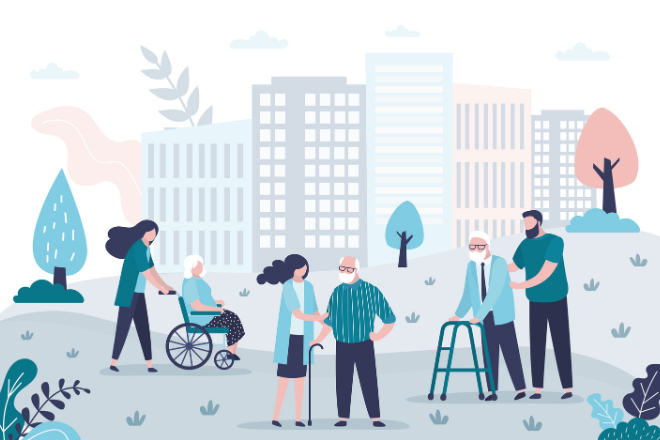
Author: Robin Kreling, Data Scientist in the Data Analysis and Science Department, Cour Des Comptes, France
The number of people over 50 receiving disability allowances increased by 36% in France between 2011 and 2019. The Court of Accounts wanted to check whether the needs of the populations concerned were being adequately met.
To this end, the Court of Accounts employed an optimal matching technique. This data science technique shows similarities in the succession of events and, thus possible causality between them. By adapting an algorithm derived from genetics, the Court of Accounts verifies possible breaks in the residence and administrative path, depending on the different situations encountered, such as whether or not people have access to specialized care or recognition of disability, and whether or not they receive care at home, etc.
The data scientists’ team was particularly inspired by the approach used by the Toulouse University Hospital for a previous survey. This methodology highlights the value of having a memory of past work and continuity of activity in the team, to capitalise on methods, practices and innovations. The effort to adjust the algorithm to the data used by the Court in this investigation consisted of choices of calculation method intended to limit the calculation time.
The data was extracted from the digital service platform ViaTrajectoire, which connects people with institutions and helps them manage queues. The available information indicated whether people had open rights for dedicated medical or social care or not. The data was pseudonymised, with different encryption keys for each department.
It should be noted that, as a result of this initial work, the Court is considering to match this data, with other administrative data for other surveys, still anonymously, thus capitalising on the knowledge gained from these databases.
An algorithm to confirm and objectify the audit team’s intuitions
The optimal matching technique applied to these data consisted of defining a similarity metric between the sequences, i.e. calculating a number giving an indication of the distance between two data sequences: while many changes are needed to transform one given sequence into another, they are considered very dissimilar and remote. If they require little or no changes, they are very close. This metric is then used to group the sequences into proximity clusters.
The grouping in clusters of typology of administrative and residential paths confirmed the intuitions of investigators and refined their understanding of the administrative, care and individual residential paths. For example, 12% of individuals in the 45- to 50-year-old sample are grouped in cluster 2. The persons clustered in this group have an administrative recognition of a disability but do not submit any known application to an institution for a long period of time: these may include persons who refuse the recommended guidance or conduct the recognition procedure as a precautionary measure for future needs for formal assistance. Knowing and quantifying the existence of such precautionary approaches is useful for calculating indicators of the tension of accommodation solutions.
Collaboration between the audit team and data scientists is essential for the success of the audit
Exchanges between the control team and the data scientists of the Data Science and Analysis Department of the Court took place as soon as the feasibility note was drawn up, prior to the initiation of the investigation. This eased collaboration and facilitated the operation of the bases during the investigation. In particular, the audit team had identified very well the databases useful for the investigation. Its exchanges with data scientists were weekly and made it possible to produce indicators requested by the auditors, to point out unanticipated situations (like preponderance of some less visible handicaps) and highlight shortcomings in administrative databases.
Reading graphs: in X-axis, the months and years (sequences of 5 years complete, from 0-01 to 5-12); in Y-axis: the proportion of cluster observations, which ranges from 0 to 1. Each cluster has a different size (the size is indicated by “Freq. (weighted n=[number]”. For example, cluster 6, we see that nearly 60% of the 3,228 people in this cluster do not experience any changes in their situation over a period of 5 years.
In this respect, it is important to stress that the audit of the data itself contributes to the audit of the steering of the public policy in question: these shortcomings, identified by the data scientists, gave rise to explicit recommendations in the report published in September 2023, which can be accessed in French here.
The resulting database is indeed very recent, but will be useful for future investigations into disability and dependency. Over time, its historical depth will increase and represent longer and more representative sequences of a life course. It will also allow causal analysis of the effects of future reforms of public policies on autonomy and inclusion of people with disabilities.
Robin Kreling, Data Scientist in the Data Analysis and Science Department
To go further:
Contact Court Data Science and Analysis Department.