Quantitative and Qualitative Methods: The Optimal Combination for Effective Audits
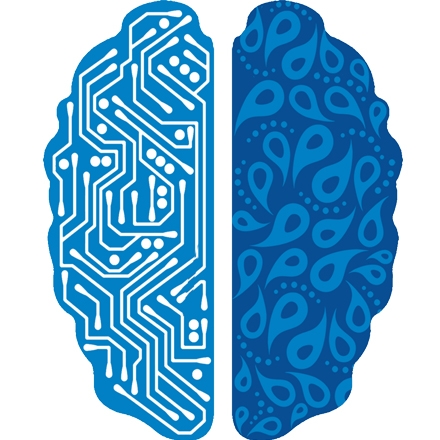
[cmsmasters_row data_padding_bottom_mobile_v=”0″ data_padding_top_mobile_v=”0″ data_padding_bottom_mobile_h=”0″ data_padding_top_mobile_h=”0″ data_padding_bottom_tablet=”0″ data_padding_top_tablet=”0″ data_padding_bottom_laptop=”0″ data_padding_top_laptop=”0″ data_padding_bottom=”50″ data_padding_top=”0″ data_bg_parallax_ratio=”0.5″ data_bg_size=”cover” data_bg_attachment=”scroll” data_bg_repeat=”no-repeat” data_bg_position=”top center” data_color=”default” data_bot_style=”default” data_top_style=”default” data_padding_right=”3″ data_padding_left=”3″ data_width=”boxed” data_shortcode_id=”dg2ang0ka”][cmsmasters_column data_width=”1/3″ data_animation_delay=”0″ data_border_style=”default” data_bg_size=”cover” data_bg_attachment=”scroll” data_bg_repeat=”no-repeat” data_bg_position=”top center” data_shortcode_id=”dz2wxhnjjp”][cmsmasters_image shortcode_id=”ss819s37sb” align=”center” link=”http://intosaijournal.org/wp-content/uploads/2019/07/Quantitative_Qualitative-Techniques_Optimal-Combo.jpg” animation_delay=”0″]19649|http://intosaijournal.org/wp-content/uploads/2019/07/Quantitative_Qualitative-Techniques_Optimal-Combo.jpg|full[/cmsmasters_image][/cmsmasters_column][cmsmasters_column data_width=”1/3″ data_shortcode_id=”ez0r31724r”][cmsmasters_image shortcode_id=”xqgcvrtqk” align=”center” link=”http://intosaijournal.org/wp-content/uploads/2019/07/Quantitative_Qualitative-Techniques_Optimal-Combo2.jpg” animation_delay=”0″]19650|http://intosaijournal.org/wp-content/uploads/2019/07/Quantitative_Qualitative-Techniques_Optimal-Combo2.jpg|full[/cmsmasters_image][/cmsmasters_column][cmsmasters_column data_width=”1/3″ data_shortcode_id=”m1mah0eju”][cmsmasters_image shortcode_id=”7wnt31qkym” align=”center” link=”http://intosaijournal.org/wp-content/uploads/2019/07/Quantitative_Qualitative-Techniques_Optimal-Combo3.jpg” animation_delay=”0″]19651|http://intosaijournal.org/wp-content/uploads/2019/07/Quantitative_Qualitative-Techniques_Optimal-Combo3.jpg|full[/cmsmasters_image][/cmsmasters_column][/cmsmasters_row][cmsmasters_row][cmsmasters_column data_width=”1/1″][cmsmasters_text]
by Oliver M. Richard, Chief Economist and Director, Center for Economics, U.S. Government Accountability Office
The creation of the International Organization of Supreme Audit Institutions (INTOSAI) Working Group on Big Data speaks to the increased attention to enhanced data analytics. While much consideration has focused on quantitative techniques, this article highlights qualitative analysis, its relevance and ability to complement quantitative methods used in Supreme Audit Institution (SAI) work.
Though they contribute differently to an audit (broadly defined to also include evaluation), quantitative and qualitative methods share a common purpose—to produce actionable information addressing the audit’s objective. Research finds the difference is more a matter of degree than type.
Qualitative methods, such as content review, interviews, focus groups and case studies, generally seek to develop an understanding and assessment of the audit’s conceptual and behavioral context. As such, they score highly on validity and inductive reasoning (moving from observation to hypothesis).
Quantitative methods, including systematic data analyses, aim for reliability and hypothesis testing. Thus, qualitative and quantitative methods are best viewed as complements in the audit process. Examining existing studies shows complementarity occurring at three levels in that qualitative analysis (1) is an essential preliminary to quantitative research, as it enables a contextual description and understanding that is instrumental in designing the appropriate audit scope; (2) supplements quantitative work (and vice-versa) by adding validation to empirical findings; and (3) can explore areas not amenable to quantitative research, such as internal control processes within an agency or program.
Together, qualitative and quantitative methods form a range of approaches that, when optimally combined, enable a SAI to decipher concepts, content and data that may exist in an audit situation. The optimal combination is case-specific, as it depends upon facts that characterize each audit.
Assessing the right methodological mix demands expertise. The U.S. Government Accountability Office (GAO) established a team—Applied Research and Methods (ARM)—of about 135 specialists with advanced degrees in 14 disciplines across the social, physical, and computing sciences who contribute independent expertise in qualitative and quantitative methods to GAO’s audit teams. ARM’s specialists work closely with GAO’s analysts to combine qualitative and quantitative techniques in GAO reports. GAO’s report, “Social Security Disability: Additional Measures and Evaluation Needed to Enhance Accuracy and Consistency of Hearings Decisions,” provides a good example of employing a combination of qualitative and quantitative tactics.
Background
The U.S. Social Security Administration (SSA) manages disability benefit programs that cover roughly 16 million Americans who receive an estimated $200 billion in benefits annually. For adult eligibility, a person must have a medically determinable physical or mental impairment that (1) has lasted (or is expected to last) for a continuous period of at least one year or result in death, and (2) prevents the person from engaging in any substantial gainful activity. The SSA received over 2.5 million disability claims in fiscal year 2016.
When a person applies, SSA makes an initial determination. Typically, when a claim is denied, the claimant may request a hearing before an Administrative Law Judge (judge). In fiscal year 2016, claimants appealed 698,000 decisions to judges, who may deny or allow a claim at a hearing. The judge’s allowance rate is the percentage of claims for which a judge allows benefits.
Stakeholders noted a variance in allowance rates across judges, and GAO was asked by the U.S. Congress to examine the extent to which allowance rates vary across judges (and what factors are associated with this variation); and the extent to which SSA processes monitor accuracy and consistency of hearings decisions.
The Analysis and Findings
GAO relied on qualitative methods to better understand SSA’s process, such as conducting a content review of applicable SSA policies and procedures; interviewing SSA officials; holding semi-structured interviews with regional chief judges; and attending hearings in selected offices (not generalizable) to conceptualize the hearings process in practice.
GAO learned, in particular, that SSA randomly assigns cases to a judge based on the claimant’s location. This practice increased the chance that factors possibly explaining differences in allowance rates across judges were similar across cases and thereby identifiable using a quantitative analysis of allowance decisions.
Quantitatively, GAO compiled information from several SSA administrative systems, federal personnel and payroll systems, and economic data (spanning fiscal years 2007-2015 that included an estimated 3.3 million claims records). Using this data, an annual allowance rate was computed for each judge.
GAO first examined the allowance rate data to substantiate concerns that motivated Congress’ request. Figure 1 shows that allowance rates vary substantially across judges, even as the average allowance rate across judges fell 15 percentage points from 2008 to 2015.
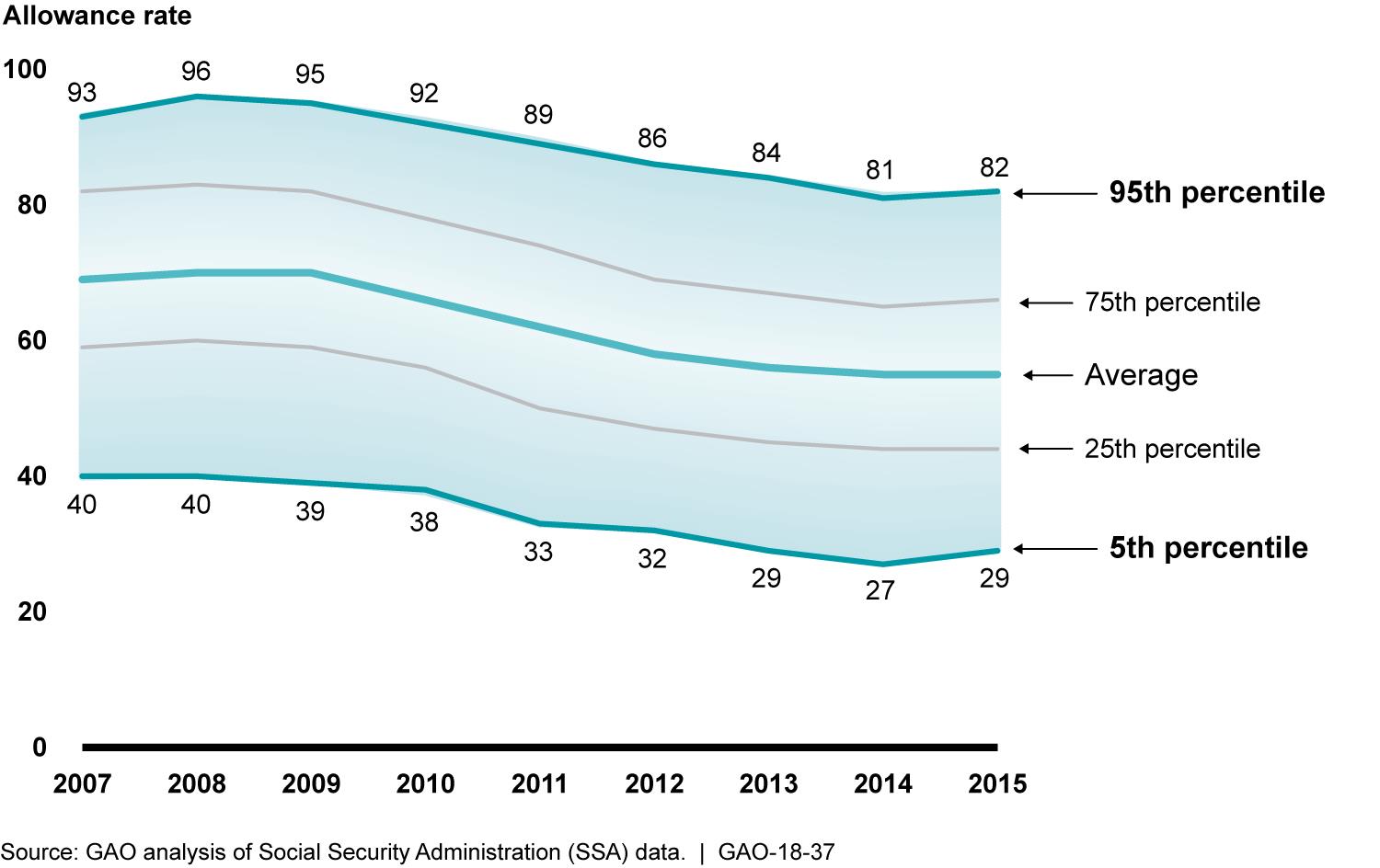
GAO engaged with SSA officials, who noted the variation is not surprising due to case complexity and judges’ decisional independence.
SSA officials also cited an expansion in quality assurance reviews and electronic data usage to monitor judges’ decisions, which should have helped lessen variation in allowance rates across judges over time. These qualitative insights raised the hypothesis that allowance rates could vary across judges due to case dissimilarity.
To test this hypothesis, GAO used individual claims data to develop and estimate a quantitative statistical model identifying factors associated with a judge’s decision to allow or deny a claim. In consultation with SSA officials, and based on a qualitative review of relevant literature, GAO controlled for certain factors, such as claimant characteristics (type of claim and demographics); judge making the decision (appointment year, prior experience); location; presence of representatives at the hearing (attorney, medical, vocational expert); and local economic conditions (unemployment and poverty rates).
As illustrated in Figure 2, GAO estimated the allowance rate varied by 46 percentage points for a typical claim depending upon the judge who heard the case. The quantitative model identified multiple factors associated with a judge’s decision, and GAO’s interpretation of these findings was supplemented by qualitative evidence. For instance, the model demonstrated claimant age mattered, as a 55 year-old claimant was allowed benefits at a rate 4.3 times higher than a typical 35 year-old claimant. SSA officials informed that this finding was consistent with SSA’s vocational guidelines, which are generally more lenient for older claimants.
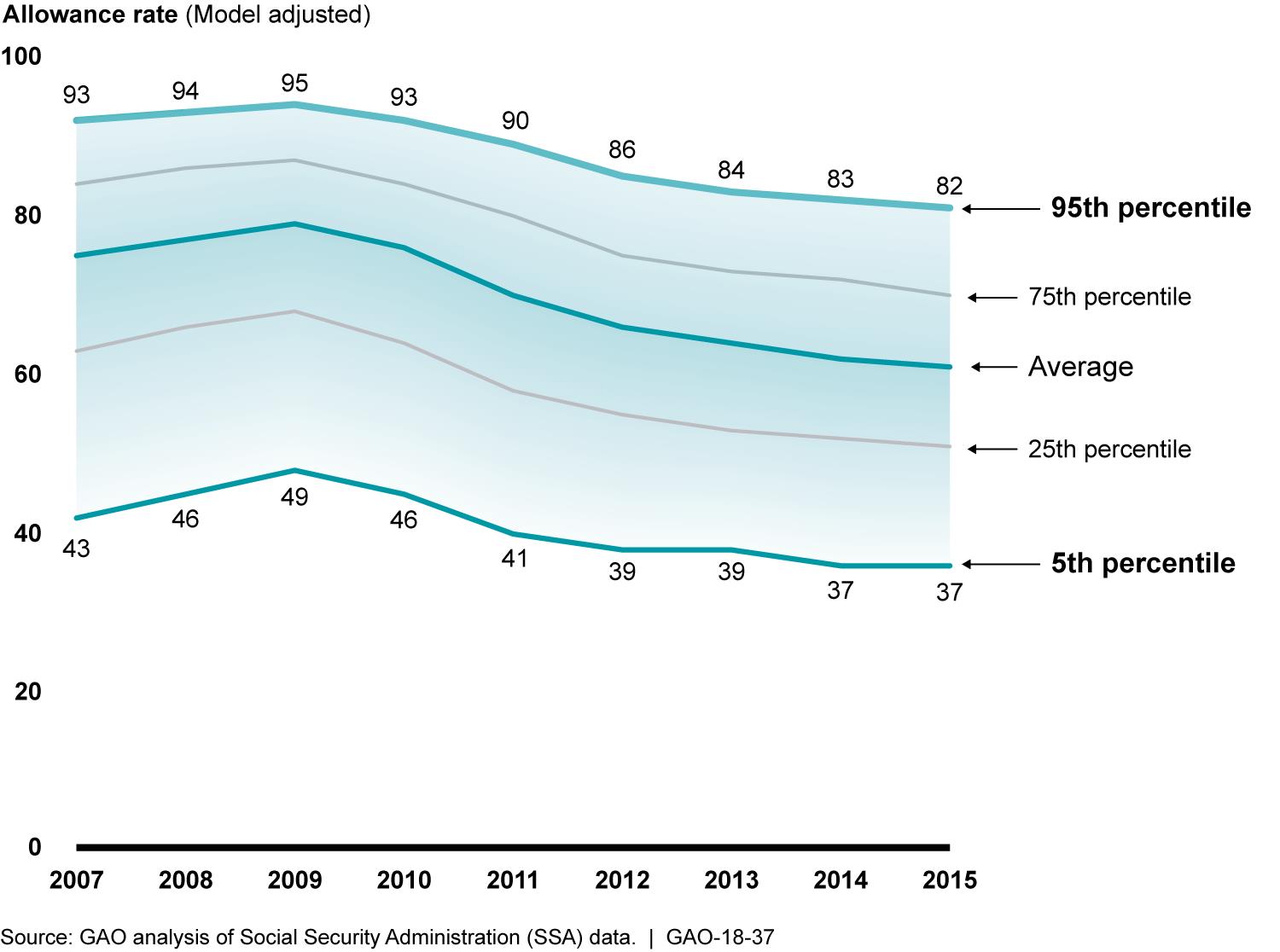
Similarly, the model found that claimants who had a representative were allowed benefits at a rate 2.9 times higher than a typical claimant with no representative. SSA officials said that, under SSA’s fee structure, representatives are only paid if the claimant is awarded benefits. As a result, representatives may tend to take cases they believe will be successful. Thus, GAO’s quantitative findings were interpreted in conjunction with qualitative evidence—further enhancing credibility. Note: A typical claim had average values on all factors GAO controlled for in its quantitative statistical model (factors related to the claimant, judge, other participants in the process, hearing office, and economic characteristics).
Finally, GAO examined to what extent SSA had processes to monitor the accuracy and consistency of hearings decisions. While this line of inquiry was an internal controls audit relying on qualitative methods, the empirical evidence developed with GAO’s quantitative model underscored the need for SSA to measure and hold itself accountable for accuracy and consistency. GAO ultimately found that SSA had not systematically considered how each of its quality assurance reviews helped the agency meet its strategic plan’s objective to improve quality, consistency and timeliness of hearings-level decisions.
Conclusion
Even as advanced data techniques become more prevalent in audit work, SAIs must maintain an audience awareness and ensure messaging is both credible and accessible.
Developing qualitative evidence that grounds quantitative findings within the audit’s conceptual and behavioral situation adds validity to audit findings and enhances the likelihood that recommendations will be implemented.
[/cmsmasters_text][/cmsmasters_column][/cmsmasters_row]