Data, Auditing & Strategy: Unlocking Knowledge in Capitalizing on Opportunities, Addressing Challenges
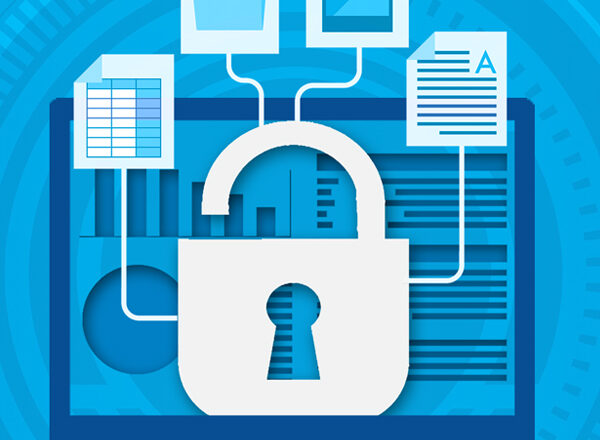
by Koen Van der Bracht, Supreme Audit Institution of Belgium
Data has become a prominent auditing buzzword—as revealed through the increased use of the term throughout the global accountability community to include the International Organization of Supreme Audit Institutions (INTOSAI) and its member Supreme Audit Institutions (SAIs).
While data opens up new possibilities for SAIs, including interesting prospects for more risk-based audits, it also presents numerous conceptual, technical and organizational challenges. This article discusses some of the opportunities and challenges data brings and provides an overview of how SAI Belgium has addressed them.
Opportunities
Data availability and data analytics have affected audit—as a field and profession—particularly as financial process digitalization has brought new techniques that allow SAIs to audit 100% of transactions. This ability increases financial audit efficiency and allows auditors to differentiate between normal and anomalous transactions. Distinguishing anomalous transactions creates additional efficiencies, as auditors no longer need to audit a large sample that may (or may not) be representative.
Performance auditing traditionally focuses on determining program economy and efficiency. However, available data (and the means to more easily analyze it in large quantities) allows auditors to directly measure program effectiveness. For instance, when auditing a poverty reduction program, SAIs can now assess actual changes in poverty resulting from government intervention.
Challenges
Data proliferation and new techniques present several challenges that can generally be divided into three categories: conceptual, organizational and technical.
Conceptual Challenges
Data availability and validity remain the primary conceptual challenges. To measure policy effectiveness, auditors may often need to rely on government databases designed for different purposes. For example, labor market participation may be derived from tax databases that can miss crucial information necessary to an audit. Prior to performing assessments using information from government databases, a study on the data’s reliability and validity may be needed. Otherwise, auditors run the risk of asking the right questions and performing the right analyses only to arrive at wrong conclusions due to data flaws.
Organizational Challenges
SAIs deciding to invest in data analytics may also need to adjust ways in which audits are performed. Obtaining the right skills is crucial and entails a thorough analysis of SAI needs, such as addressing the type(s) of data analytics desired and necessary support staff, including skills and knowledge in database administration, data protection regulations and data visualization. Additionally, SAIs should consider how these skills can be obtained, either by training current staff or recruiting new employees.
Addressing organizational setup is essential, and many options are feasible based on a continuum that includes data specialists allocated to teams only performing analyses for other audit teams to data specialists fully embedded into audit teams performing audits from start to finish and only analyzing data for individual audits.
Technical Challenges
When performing data analytics in an audit context, SAIs may face technical challenges and require new software or more robust computers.
Early consideration to necessary software provides numerous benefits: potentially minimizing costs while catering to current and future audit needs; providing a benchmark for skills; and selecting appropriate data protection solutions. New software solutions may also require high-performing computers—for each data analyst and/or for shared central servers or cloud-based solutions. These options may also depend on how data specialists are allocated. If data specialists, for instance, work on audits from start to finish, it may make sense to pool computing resources with central, shared servers or cloud-based solutions, as the amount of specialists that will do data analytics simultaneously will be limited.
Regardless of the software and hardware options, data protection will always need to be addressed. As more data are harvested, edited and stored, investments in protecting these data becomes more crucial, especially considering the personal and/or sensitive nature of the data.
The Belgian Solution
SAI Belgium’s strategic plan focuses on directly measuring policy effectiveness, which often goes hand-in-hand with data analytics. To implement this strategic plan, the SAI has employed numerous tactics, such as:
- Hiring academically trained social scientists with data skills;
- Performing a survey to detect experiences (and interest in) data analytics among existing staff;
- Organizing a global training session on the basics of data analytics; and
- Forming a “DataLab” discussion group that helped spread data analytics agency-wide through monthly meetings incorporating organized training, advice and assistance from data specialists.
SAI Belgium chose to allocate data specialists to audit teams, where they perform audits from start to finish. However, through DataLab, data specialists also support teams that have no data analytic specialists.
Because several software packages are used simultaneously, SAI Belgium continues to face technical challenges—a result of employees with a diverse background using existing software combined with the relatively new use of data analytic techniques.
Currently, SAI Belgium is investing in high-performance, centralized computers and developing a Structured Query Language warehouse to store recurrent financial data flows from administration. Cloud-based solutions are also being investigated, and the organization’s Data Protection Officer has established a data protection policy.
Conclusion
Data availability and techniques can offer useful prospects for new audit types. While SAI Belgium has fully embraced these possibilities, some lessons learned include the need to (1) thoroughly consider a data analytics strategy; and (2) consider potential conceptual, organizational and technical challenges.
While SAI Belgium experienced all of the challenges outlined in this article, the creation of DataLab proved crucial to addressing these challenges, particularly as the group provided a forum to brainstorm strategies to overcome all challenges and capitalize on all possibilities.